In the 16th century, the rapid growth of overseas trade sparked a heated debate in the artistic world. Whereas fresco painting - a technique of embedding pigment onto walled surfaces - was the premiere artistic method at the time, a growing supply of canvas from the maritime industry offered artists a cheaper alternative. In the century-long struggle between creative factions, canvas would prove victorious, becoming the medium of choice for masterpieces such as the da Vinci’s Mona Lisa and van Gogh’s Starry Night.
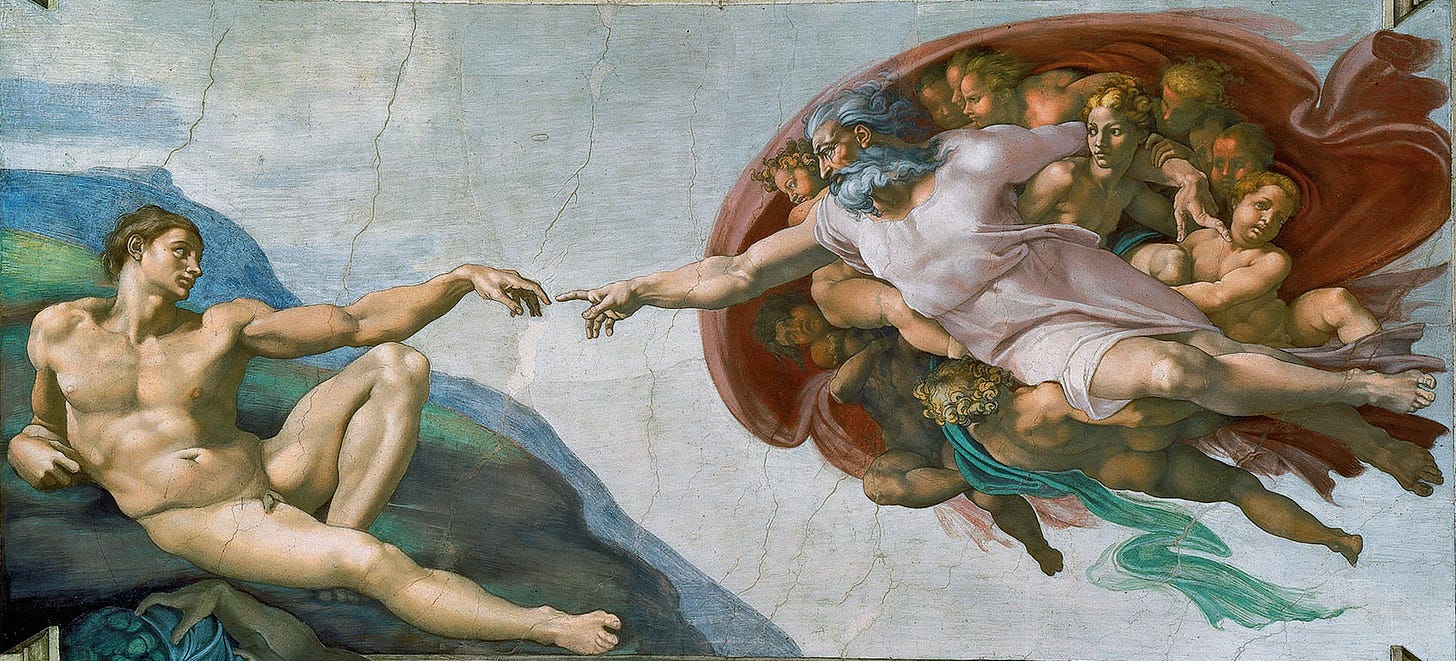
With the bias of hindsight, we forget that early canvas paintings were made amidst a backdrop of deep criticism. At the time, true art was made to be timeless; embedded along the ceiling of a cathedral or the walls of a palace. Canvas had entirely opposite properties: it was cheap, lightweight, and entirely detached from its surroundings. It’s dominance changed the very act of creation. But what felt like a loss of culture at the time turned out to be a massive gain. Canvas made art accessible, bringing even more voices and perspectives into the artistic sphere.
Today’s rejection of another modern medium is only an echo of this same debate. At the dawn of art's digital era, we manage to find something deeply troubling about the efficacy of generative ML models. For many, it feels like the beginning of the end for art. But this response is a product of our collective shock; a protective instinct when change happens too fast.
The truth is we’re converging on a new paradigm of creativity. Art won’t disappear, but adapt. ML-enabled tools will empower every person online with god-like creative abilities. Entire industries - entertainment, marketing, computer graphics - will be transformed and redefined. This change will be a dramatic one, but to embrace it we must overcome our disbelief. Creative ML is here, and it’s only getting better.
Universal Creativity (For Free!)
For many of us, it's fairly surprising that creativity would be one of the first bastions to fall to machine learning. That's because we generally think of ML as being good at answering prediction-type problems, such as: Does this chest X-ray have a tumor in it? Does this picture contain a cat? This is the type of narrowly applied AI that we have come to expect.
Except in 2014, ML researchers discovered something the public is just beginning to understand. We can ask these same questions in reverse! For instance: “Show me a chest X-ray” or “Show me a picture of a cat”.
Instead of making models that deduce, researchers learned to make models that produce. By training a model to generate accurate representations of image or text, machine learning could become part of the creative process, opening an entirely new category of research.
This expansion in capability is deeply impactful, because like all software, machine learning benefits from the laws of digital abundance. This framework, originally found in The World After Capital, helps us understand the economic impact of digital products and services. It’s two core principles are:
The law of zero marginal cost, and
The principle of universal computation
We experience zero-marginal cost as the relative ease with which we can cheaply share digital information. Even the best AI models can be published on GitHub and downloaded by a teenager - for free. Just as you can access nearly any textbook or movie online.
The idea of universal computation relies on the fact that computers are ‘universal machines’. They can be programmed to perform any task (which is mathematically proven by the way). This can be (intuitively) extended to infer that ML models themselves can be trained to perform nearly any task. A statement which so far seems to hold up!
All this means that when a step-function improvement in capability -or an entirely net new capability appears- it’s time to pay attention. Because that new functionality will become ubiquitous. Within days of its announcement, it will be shared across the internet at near-zero cost. Which is why, in short order, the application of creative ML models will become embedded into everyday products.
The Hidden Abilities of Creative ML
What if I told you that DALLE-2 was only the tip of the iceberg? Perhaps even the tip of the tip of the iceberg. Even with the public awareness that image-generation models have already received, I sense that we are unprepared for the disruption coming to creative fields. For this reason, I want to use this article to explore different applications of creative ML.
Simulation
Digital simulation, in particular the simulation of fluids, is an incredibly compute-intensive process. As one would imagine, trying to recreate the interaction of billions of particles is really really hard. But it’s essential to creating the high-quality graphics used in blockbuster movies, and even more necessary for use in industrial applications like aerospace engineering. For this reason, both industry and Hollywood have spent decades refining software stacks that accurate and efficiently render such simulations.
Research has shown that it’s possible to use ML predict the behaviour of particles, leading to immense speed ups in render time and reduction in file sizes. In the simulation below, NVIDIA’s AI-integrated software uses 18x less data and processed at 1.7x faster speeds than the leading simulation software.
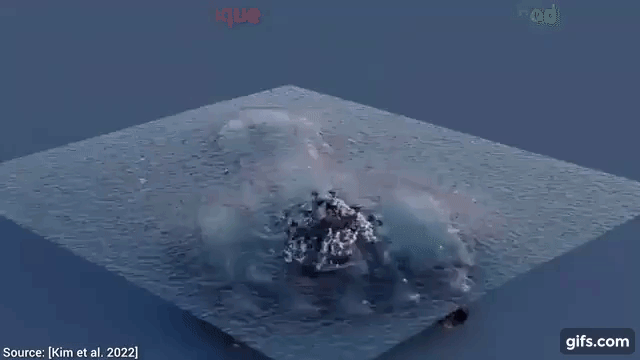
Increasing render time and decreasing the data footprint of a simulation is fundamentally cost-saving, but it also makes this technology more accessible. With models this performant, even amateur video-editing software can offer high-quality effects to their users.
Animation
In video games, user are constantly interacting with non-player characters (NPCs). To keep this experience immersive, animators need to program NPC movements to be as realistic as possible. This time-consuming process is repeated for every manner of being, from human or animal companions to mythical foes and sci-fi enemies.
In a paper titled Deep Learning for Character Control, an AI model was trained to animate both bipedal and quadrupedal characters. It went a step further and was able to animate the character’s interaction with their environment (which you can see in the GIF below). These AI-controlled characters could sit in furniture, maneuver around obstacles, and pick up objects. Imagine the cost-savings this will bring (and likely already has brought) to game development!
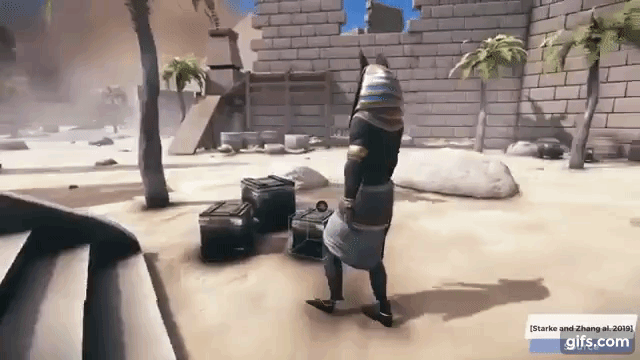
Character-Driven Storytelling
While creative ML can help us generate an image of any scene with just a few words, it’s often the case that we need to create more than a single picture. This introduces the problem of ‘subject-level consistency’. To tell a story, your main character needs to continue to look like your main character. And while it’s possible to make high-quality characters with one-off image-generation, a lack of character consistency holds this technology back from more advanced use cases.
Using the DreamBooth model released in August 2022, the YouTube channel Corridor Digital was able to create a consistent multi-character story using reference images of their actual employees. Their video blew. my. mind. They were able to create dozens of illustrations (a few depicted below) that could have easily been the pages of a children’s picture book.
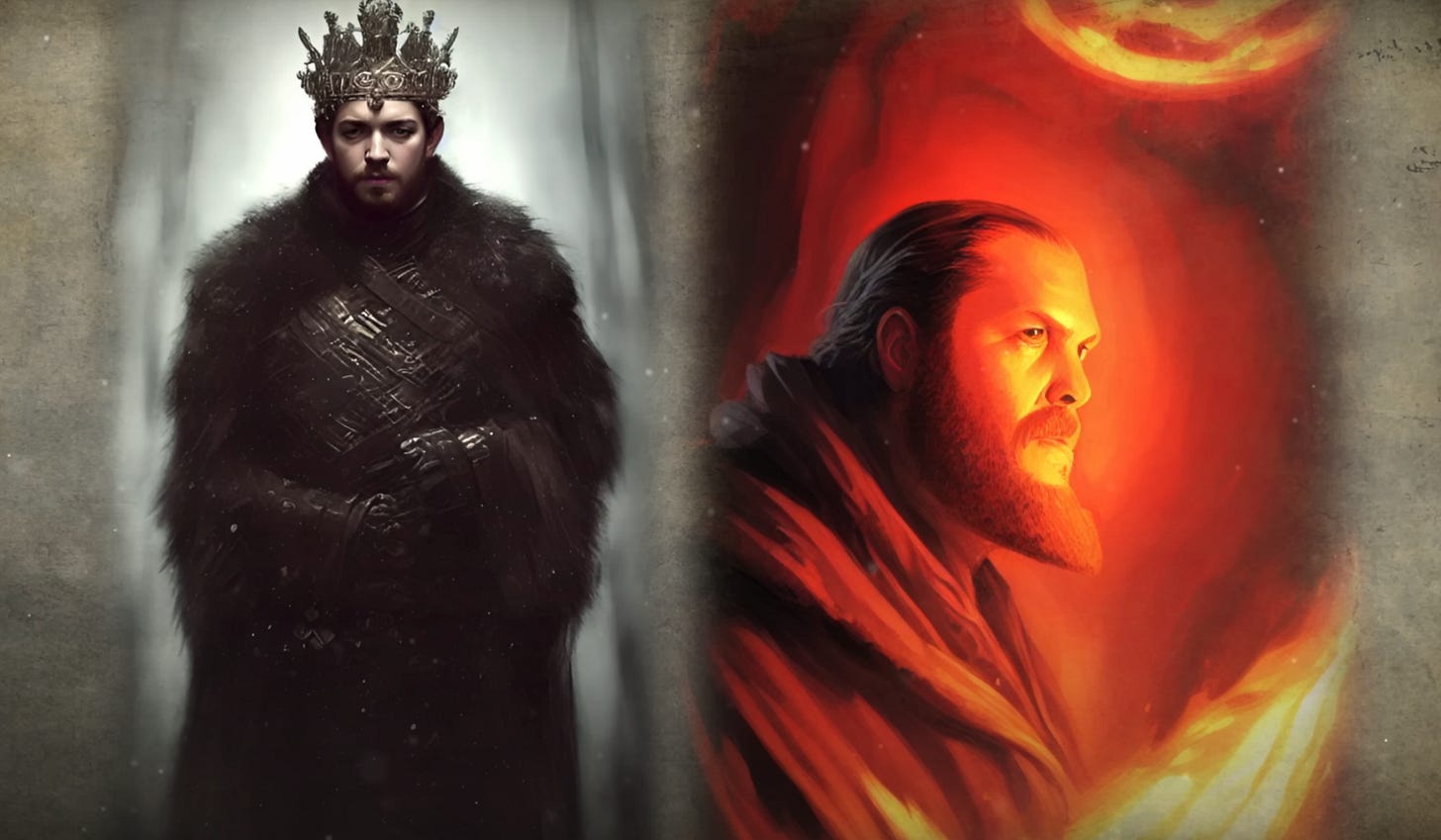
As these models evolve to generate not just images, but videos, it feels inevitable that we will see entire TV series and feature-length movies that are produced by a single individual. These models are already able to be used for storyboarding and earlier steps in the creative process.
Product Marketing
DreamBooth’s technology can be applied to more than human characters. By using reference images of an object, it’s possible to depict a product in arbitrarily generated settings while maintaining subject-level consistency. To me, this feels like the world’s most powerful marketing tool. With a mere handful of images you can depict your product in any scene without having to go there physically - or without that scene having to actually exist!
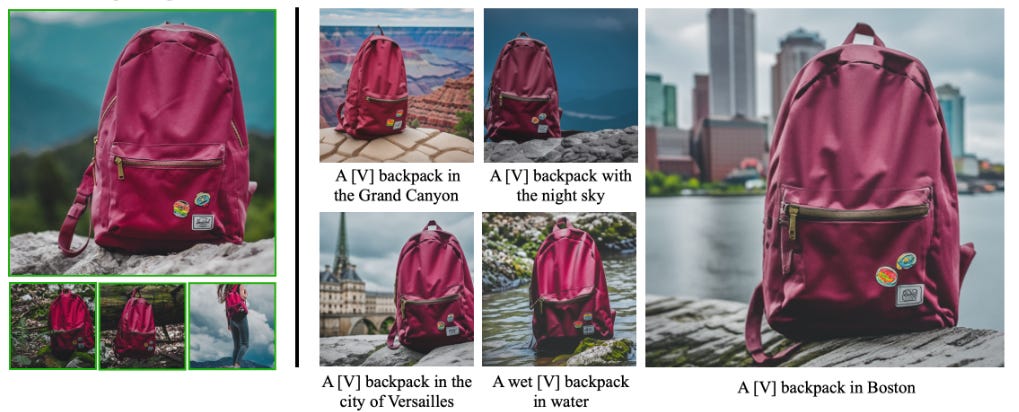
This technology is likely to start an era of hyper-personalized advertising. With knowledge of search history or data from internet cookies, it’s possible to customize an object’s setting towards individual consumer preferences. If you’re planning a trip to Europe, the backpack above might appear next to a famous tourist sites - perhaps one at the very destination you'll be flying into. Or more whimsically, if you’ve recently played a video game, this backpack could be worn by the game’s main character. The possibilities are endless.
Conclusion
With such stunningly visual results, it’s easy take for granted that these technologies will become universal. But the story of progress is never so simple.
There are yet more hurdles to overcome to enable these far-flung visions, and business models to discover (or re-apply) to harness the economic impact of this technology. Regulation, too, can make or break the entire industry. Especially because of ML’s dependence on lax policies around intellectual property as it pertains to training data. What’s more, is we’ve yet to discuss how creative work in our economy will reconfigure themselves around these powerful new tools. How will traditional ‘creatives’ adapt? What new roles will emerge, and even thrive, in a world of infinitely leveraged creativity?
It feels to me like the makings of another article 😉
If you enjoyed this article consider subscribing for weekly content on the technology that will shape our future!
If you really enjoyed this article consider sharing it with an intellectually curious friend or family member!
Let me know what you thought about this piece on Twitter.
With gratitude, ✌️
Cooper